The artificial intelligence (AI) divide between industrialized and developing countries is not just about being able to access and use new technologies. It’s also about governments and local enterprises around the world being able to create their own AI tools, to both benefit from their potential and better mitigate their risks.
A key issue with AI that needs to be addressed is equitable ownership of AI systems, and access to benefits. This question is in line with the challenge posed by the digital divide, as accelerating digitization has widened the social and economic gap between those who can access the internet, and those who cannot.
Policies related to standardizing and regulating artificial intelligence are defined by initiatives from civil society, private sector companies and national governments. However, since 2021, there have been increasing efforts towards global governance. Most recently, a United Nations Multistakeholder Advisory Body on Artificial Intelligence was announced.
Model approaches
Many of the risks that have been observed in AI — discrimination, stereotyping, general lack of appropriateness for different specific geographic and cultural contexts — are due to the use of computational modelling by AI systems. Computational modelling provides a simplified representation of reality which is then used to make predictions.
The use of models has a rich lineage within western thought traditions. It evokes Plato’s theory of forms, which suggests that the material realm is a pale imitation of the realm of concepts or forms, which represent the intangible essence.
Today, the word “model” is associated with a wide spectrum of ideals that we should aspire to — the term “role model” comes to mind. Even the occupation of a fashion model has evolved into an archetype to be mirrored, with professional models seen as representing an ideal of beauty.
(Shutterstock)
The problem with models
In the 1980s and 1990s, there was much criticism of the economic models promoted in developing countries by international financial institutions. These were criticized notably by American economist and Nobel laureate Joseph Stiglitz for using a “one-size-fits-all” approach that had adverse effects on the economies of several countries.
Not only are the financial assets connected to AI concentrated in a few nations, but the ways of thinking about AI itself are also centralized. Most large AI models are crafted by a few companies in the United States and China, using training data that largely reflects their own culture, and often by developers who lack an understanding of local circumstances beyond their own.
For example, while over one-third of the global population uses Facebook, the algorithms that steer it — such as content recommendations, contact suggestions and facial recognition — were primarily devised using data accessible to American developers.
This means that like many other flawed models in the past, biased AI models may not accurately reflect the context in which they are being applied, because the underlying data used to construct them is itself unrepresentative.
Over the years, the biases included in these models have become apparent. These issues range from an inability to accurately identify individuals with diverse skin tones, to producing discriminatory outcomes for different groups and the exclusion of specific populations.
Such outcomes are not entirely unexpected, considering the historical and geopolitical context of the impact of models. As we’ve observed, models of various sorts — whether they pertain to economics or technology — can be potentially harmful if they are misapplied.
Localizing models
AI has taken the concept of models quite far — the social and economic potential is enormous, but only if the models are calibrated properly. A pivotal solution to this revolves around the idea of model localization: the most fitting and relevant AI systems should be grounded in local contexts.
This requires decolonizing data and AI. This sentiment also mirrors the growing inclination toward digital sovereignty, enabling nations to independently manage their digital infrastructure, hardware, networks and facilities.
My research on transplantation, adaptation and creation (TAC) approaches studies the uses of AI in public health globally. During the COVID-19 pandemic, AI models were used in planning health services, communicating with the public and tracking the spread of the disease.
The pandemic brought into sharp focus variations in geographic and cultural landscape that required a nuanced approach to modelling. The TAC approach examines each application of AI while considering whether it was transplanted directly from another context without modification; adapted or modified from another model; or created locally.
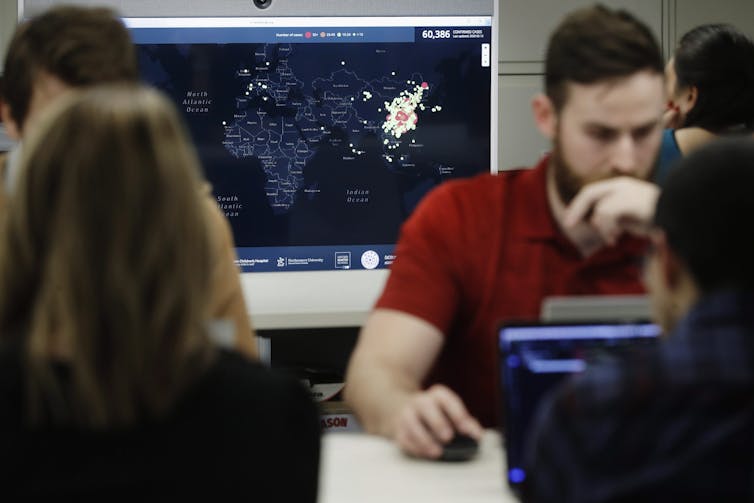
(AP Photo/Steven Senne)
Understanding the challenge
Addressing the global AI divide requires understanding that we are dealing with, at least in part, a modelling problem, similar to past modelling problems. One of the challenges that policymakers will therefore face is assessing how to ensure that AI models can be developed and deployed by as many countries as possible, allowing them to benefit from their potential.
Solutions, such as applying the TAC framework, moves us towards model localization, ensuring that applications of AI adapt to diverse global realities as much as possible.