To most effectively deliver aid to alleviate poverty, you have to know where the people most in need are. In many countries, this is often done with household surveys. But these are usually infrequent and cover limited locations.
Recent advances in artificial intelligence (AI) have created a step change in how to measure poverty and other human development indicators. Our team has used a type of AI known as a deep convolutional neural network (DCNN) to study satellite imagery and identify some types of poverty with a level of accuracy close to that of household surveys.
The use of this AI technology could help, for example, in developing countries where there has been a rapid change of land use. The AI could monitor via satellite and potentially spot areas that are in need of aid. This would be much quicker than relying on ground surveys.
Plus, the dreamy images our deep learning model has produced give us a unique insight into how AI visualises the world.
Authors/Google, CC BY
A DCNN is a type of advanced AI algorithm commonly used in processing and analysing visual imagery. The “deep” in its name refers to the multiple layers through which data is processed, making it part of the broader family of deep learning technologies.
Earlier this year our team made an important discovery using the DCNN. This network was initially trained on the vast array of labelled images from the ImageNet repository: a huge pictorial dataset of objects and living things used to train algorithms. After this initial phase, where the network learned to recognise various objects, we fine-tuned it using daylight satellite images of populated places.
Our findings revealed that the DCNN, enhanced by this specialised training, could surpass human performance in accurately assessing poverty levels from satellite imagery. Specifically, the AI system demonstrated an ability to deduce poverty levels from low-resolution daytime satellite images with greater precision than humans analysing high-resolution images.
Such proficiency echoes the superhuman achievements of AI in other realms, such as the Chess and Go engines that consistently outwit human players.
After the training phase was complete, we engaged in an exploration to try to understand what characteristics the DCNN was identifying in the satellite images as being indicative of “high wealth”. This process began with what we referred to as a “blank slate” – an image composed entirely of random noise, devoid of any discernible features.
In a step-by-step manner, the model “adjusts” this noisy image. Each adjustment is a move towards what the model considers a satellite image of a more wealthy place than the previous image. These modifications are driven by the model’s internal understanding and learning from its training data.
As the adjustments continue, the initially random image gradually morphs into one that the model confidently classifies as indicating high wealth. This transformation was revelatory because it unveiled the specific features, patterns, and elements that the model associates with wealth in satellite imagery.
Such features might include (but are not limited to) the density of roads, the layout of urban areas, or other subtle cues that have been learned during the model’s training.
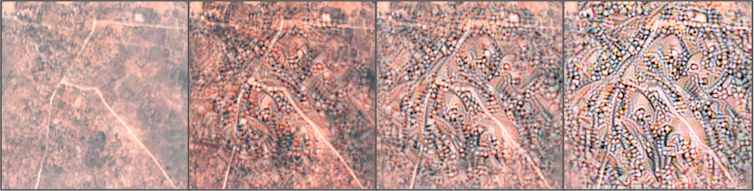
Authors/Google, CC BY
The sequence of images displayed above serves a crucial purpose in our research. It begins with a baseline satellite image of a village in Tanzania, which our AI model categorises as “poor”, probably due to the sparse presence of roads and buildings.
To test and confirm this hypothesis, we progressively modify each subsequent image in the sequence, methodically enhancing them with additional features such as buildings and roads. These augmentations represent increased wealth and development as perceived by the AI model.
This visual progression shows how the AI is visualising “wealth” as we add things like more roads and houses. The characteristics we deduced from the model’s “ideal” wealth image (such as roads and buildings) are indeed influential in the model’s assessment of wealth.
This step is essential in ensuring that the features we believe to be significant in the AI’s decision-making process do, in fact, correspond to higher wealth predictions.
So by repeatedly adjusting the image, the resulting visualisation gradually evolves into what the network “thinks” wealth looks like. This outcome is often abstract or surreal.
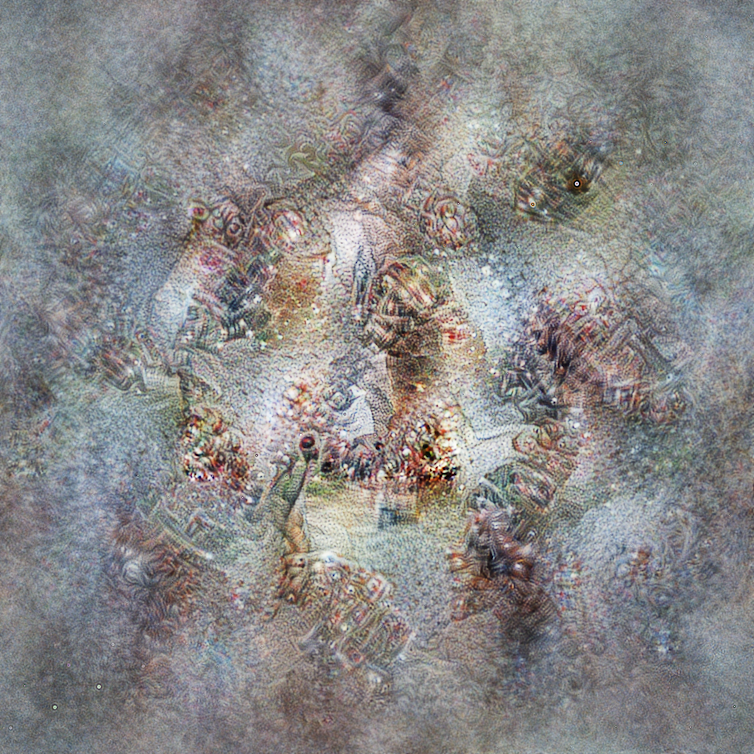
Authors, CC BY
The image above was generated from a blank slate when we asked the DCNN what it associated with “high wealth”. These images have an ethereal quality and don’t closely resemble typical daytime satellite photos. Yet, the presence of “blobs” and “lines” suggests clusters of homes interconnected by roads and streets. The blue hue might even hint at coastal areas.
Dreamy images
Inherent in this method is an element of randomness. This randomness ensures that each attempt at visualisation creates a unique image, though all are anchored in the same underlying concept as understood by the network.
However, it is important to note that these visualisations are more a reflection of the network’s “thought process” rather than an objective representation of wealth. They’re constrained by the network’s training and may not accurately align with human interpretations.
It is crucial to understand that while AI feature visualisation offers intriguing insights into neural networks, it also highlights the complexities and limitations of machine learning in mirroring human perception and understanding.
Understanding poverty, particularly in its geographical or regional context, is a complex endeavour. While traditional studies have focused more on individual aspects of poverty, AI, leveraging satellite imagery, has made significant strides in highlighting regional poverty’s geographical patterns.
This is where the real value of AI in poverty assessment lies, in offering a spatially nuanced perspective that complements existing poverty research and aids in formulating more targeted and effective interventions.